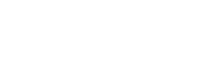
PhD Defense: Active Vision for Embodied Agents Using Reinforcement Learning
4 June 2024
Abstract: Similar to humans and animals, embodied agents can only partially observe their surroundings from their egocentric perspectives at any moment. An embodied agent, when presented with a specific objective, is expected to proactively collect the necessary information to accomplish the goal. Nevertheless, the issue of insufficient observations could arise, posing challenges in the development of the active visual system.
This dissertation approached the issue from the perspective of reinforcement learning (RL), aiming to answer three specific research questions: (i) How to endow disembodied models with the capability of information sufficiency evaluation? (ii) How to model active-vision embodied agents using neural networks and optimize the active vision control policy using RL? (iii) How to stabilize the RL process of the active vision control policy to make the training more efficient? To answer these questions, this dissertation presented practical methodologies, novel assessment tasks, theoretical and empirical analysis, and comprehensive discussions.
Date and Time: Tuesday, 04 June 2024, 14:00 CET.
Room D-220, Informatikum Campus, Vogt-Kölln-Str. 30, 22527 Hamburg.